When I Stat Scouted the Blue Jays farm earlier this month (1, 2), I simply perused minor league leaderboards sorted by statistical indicators like Age, BB%, ISO, etc. and plucked some names from the top of the pile. That's an admittedly lazy way of identifying projectable minor league talent, but the goal there was simply to identify some statistically interesting Blue Jays prospects so I could write about them, and not to place those prospects within any particularly informative minor league context.
This is the slightly less lazy version of that endeavour - I will use the information that we know correlates with future MLB success to calculate cumulative Z-scores for players in the minor leagues, resulting in a leaderboard of each leagues' top statistical prospects. This is nothing new, of course, but it's at least a bit more rigorous than simply sorting leaderboards and eyeballing players.
We know which statistics correlate with MLB success because of Chris Mitchell and KATOH. (Unfortunately we won't be getting a KATOH top 100 for 2019 because Chris Mitchell left the public game.) It's also largely common sense, I think. Generally speaking, for hitters, some easily-accessible core indicators that correlate with MLB success are Age, K%, BB%, ISO, BABIP, and SB%. These aren't equally important though - BB% for example has less predictive significance than K% and perhaps almost no projectable significance at all in the lower minors.
KATOH was a projection system that regressed and weighed those inputs appropriately and translated the inputs into MLB WAR projections. My technical skills are limited to exporting a .CSV and mucking around in an outdated version of Excel, so I won't be attempting to replicate KATOH. All I am doing is producing a lazy, unweighted leaderboard using most of the KATOH inputs.
Method
The first step is to arbitrarily pick a PA cutoff. I wanted to pick a cutoff that is inclusive without being so low that a lot of the input statistics are not even close to stable. For Low-A, I settled on 100 PA as the inclusion level. For reference, a full short-season for a hitter can be around 300 PA.
If you don't know what a Z-score is, it's how many standard deviations a figure is from the mean in the sample. Here is an illustration using Otto Lopez of the Vancouver Canadians. His BB% was 12.6% in 2018 and the average BB% of my sample was 8.76%. The standard deviation of BB% in my sample was 3.41%. Otto Lopez' Z-score for his walk rate was about +1.12, in other words, his walk rate was a little over one standard deviation better than his peers in my sample.
My list simply displays cumulative Z-scores for a player's Age, K%, ISO, and BABIP. If a player is better than average by a significant degree and/or across the board for those stats, their cumulative Z-score will take them to the top of the list. Simple!
Shortcomings and Discussion
BABIP - Batting average on balls in play might seem like a weird inclusion to you, since at the MLB level a player's BABIP is often talked about when figuring out if a player was lucky or not. We do know that certain players can consistently put up high BABIPs, due to hitting the ball hard, running fast, or both. We also know that minor league BABIP correlates with eventual MLB success, and this is probably for similar reasons - players who sting the ball and/or run fast will obviously have high minor league BABIPs. Essentially, BABIP is a proxy measure for either batted ball velocity or foot speed/athleticism.
SB% - I decided not to include SB% in my table largely to simplify the task, but also because a) SB% was not a statistically significant correlate with MLB success across all minor league levels according to Mitchell, and b) I think, as a matter of common sense, speed information is somewhat implicit in BABIP. Note that the "SB%" Mitchell uses in KATOH is not your traditional stolen base success rate. Instead, it is the propensity for a player to run when they are on first base [SB% = (SB+CS) / (Singles + Walks + HBP)]. This would correlate loosely to MLB success because it is a proxy measure for speed/athleticism, which is better than traditional SB% which might erroneously benefit a slow but smart baserunner.
BB% - We know from Mitchell that BB% is less important than the other inputs and, like SB%, not a statistically significant correlate with MLB success across all minor league levels. Mitchell told us in 2014 that MiLB BB% is only generally correlated with MLB success largely because it is collinear with ISO. Therefore, I did not include BB% in the cumulative Z-Scores. When I do this for AA and AAA, I will include BB%.
Age - We know anecdotally that age vs. level is important because we have seen old players destroy minor league levels but not develop into major leaguers and young players simply hold their own at advanced minor league levels on the way developing into MLB stars. In the lower minors, a matter of months can actually be important when it comes to age. The leaderboards I exported only displayed ages as whole numbers. The player scores for age are imperfect, a player who is 20 and 4 months gets the same score as one who is 20 and 10 months. Players born only a few weeks apart could be deemed to have played the season as 19 and 20 based on Fangraphs' leaderboard display.
Position - I believe KATOH did not actually include positional information and Mitchell admitted that it underrated players who manned a premium position. It would be cool to be able to easily pull positional information and apply some sort of adjustment to these scores, but that's above my zero dollar pay grade.
Playing Time - Obviously, an elite K% across 300 PA should be valued more than the same K% produced in 100 PA. I make no effort here to correct for or weigh playing time, aside from setting the PA cutoff for my initial export.
No weighting - All I am doing is making a decision about which stats to use and then weighing them equally. An attempt to reproduce KATOH would do something more (and something very different) - it would use regression to apply appropriate weighting to the utilized stats. When I use BB% for the AA, AAA, and maybe A+ lists, I might slice the Z-score for it in half so it is arbitrarily less important than Age, K%, ISO, and BABIP.
Results - Northwest League
Here is the top 20 in the NWL, featuring two Blue Jays prospects:
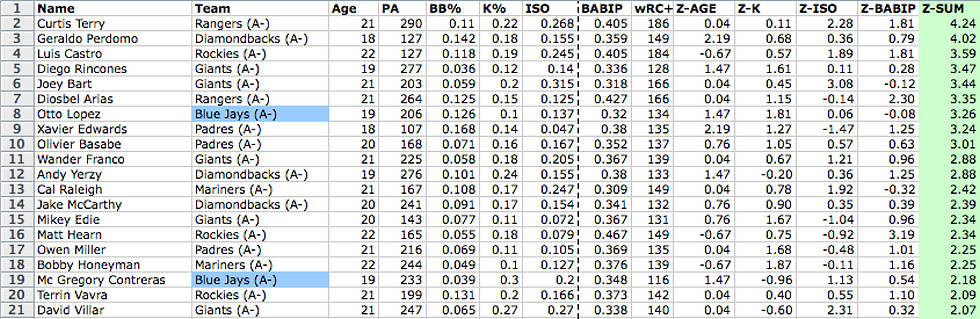
When I more lazily dug up interesting Blue Jays prospects a few weeks ago I said that Otto was the only Vancouver Canadian worth mentioning, but this method also brings up the beautifully-named Mc Gregory Contreras who I previously ignored due to an ugly BB% and an equally ugly K%. Contreras gets a positive cumulative score due to his age and propensity for stinging the ball.
Note that when I talked about Otto Lopez a few weeks ago (and as a calculation example further up in this article) I highlighted his BB%, but his Z-Score here ranks him 7th in the NWL while ignoring that high BB%. Otto is young, he makes contact, and he doesn't have any significant inability to get extra base hits. We also know he can play almost any position, so we can give him some brownie points. I like him a bit more now.
It's also nice to see North York's Andy Yerzy developing in a very real sense.
Results - New York Penn League
Here is the same top 20 for the other low-A league, the NYPL:

I don't have much to add about these names, but please add a comment below if you have any information to share!
Tyler Freeman is really separated from the pack here and he also played most of his games at SS this year, so he's certainly going on my 2019 MiLB watch list.
Here is a link to the complete spreadsheet for all 271 qualifying low-A players: THE LINK
Please give us/me a shoutout or cross-link if you use the info anywhere.
In the future, I'll work my way up the minor league ladder and post the 2018 lists for all other leagues. Or I might get some of my podcast co-hosts to do it. Stay tuned in.

Thanks for reading Stat Scouting the Minors: Z-Scores for Low-A Hitters by Nick Hill. If you have any questions or comments relating to this article, we encourage you to leave them below. For all general inquiries, we can be reached at the following:
Twitter https://twitter.com/radio_scouts
Commentaires